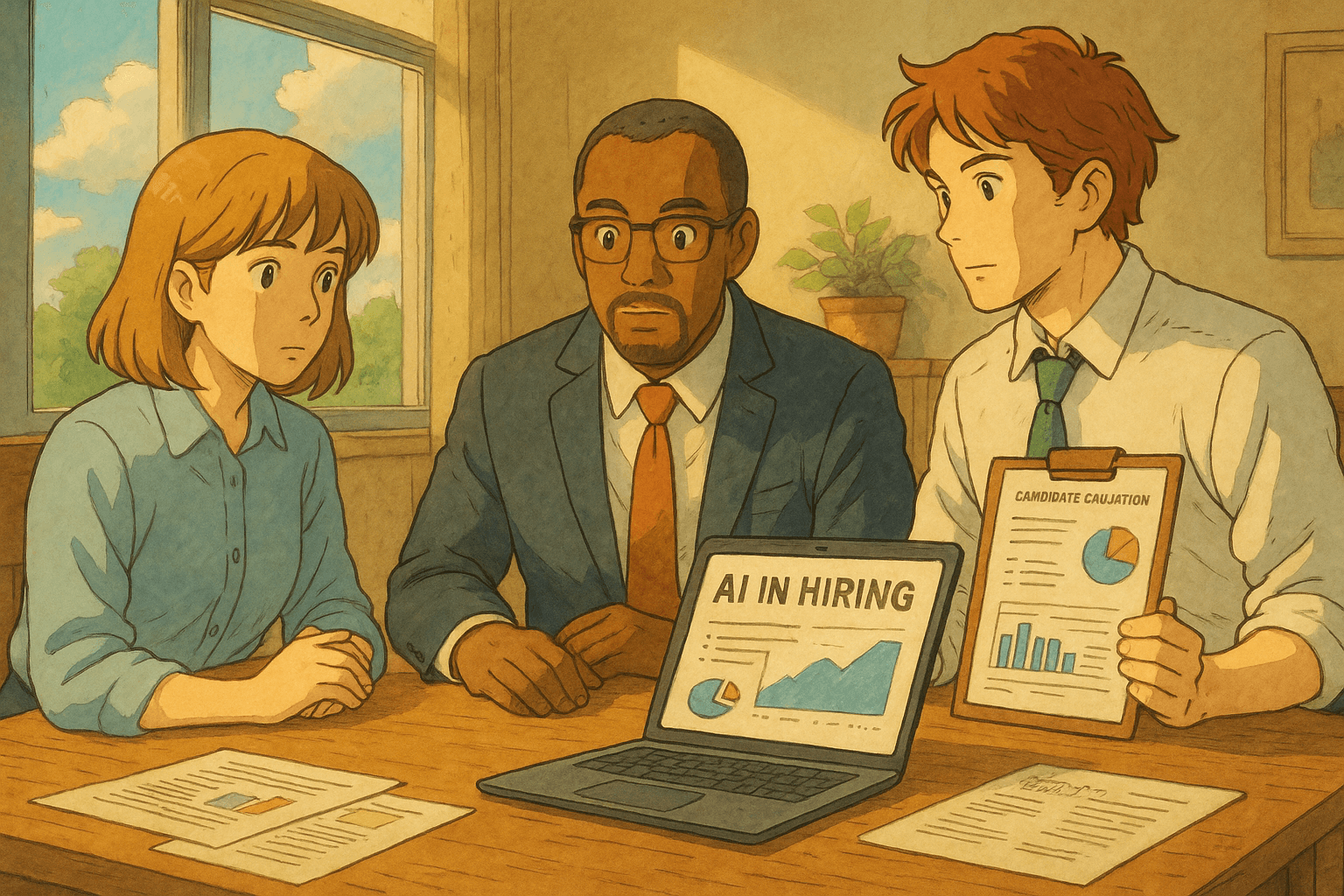
6 Ways AI Transforms Recruitment: Building Efficient and Equitable Hiring Practices
In today's competitive talent landscape, organizations face unprecedented challenges in identifying and securing the right candidates. With application volumes reaching record highs and recruitment teams operating with limited bandwidth, traditional hiring methods often prove insufficient to meet modern demands. Artificial intelligence has emerged as a powerful solution, offering practical applications that go beyond buzzwords to deliver tangible improvements across the recruitment lifecycle.
This article explores six evidence-based approaches to integrating AI into the hiring process, providing recruitment professionals with actionable strategies to enhance efficiency, reduce bias, and improve hiring outcomes.
1. AI-Powered Resume Screening: Transforming Volume into Value
The modern recruitment challenge often begins with managing overwhelming application volumes. For many organizations, a single job posting can attract hundreds or even thousands of applications, creating significant bottlenecks in the screening process.
The Challenge Quantified:
Research from talent acquisition leaders indicates that recruiters spend approximately 23% of their working hours screening resumes—yet traditional manual methods are prone to inconsistency and oversight. Studies have shown that recruiters typically spend just 7.4 seconds reviewing an individual resume, creating substantial risk of overlooking qualified candidates.
How AI Transforms the Process:
Advanced natural language processing systems now offer sophisticated analysis capabilities that extend far beyond basic keyword matching:
- Contextual Understanding: Today's AI can differentiate between a candidate who "led development of a machine learning algorithm" versus one who simply "used machine learning tools," providing deeper insight into experience levels.
- Skill Inference and Mapping: Modern systems identify relationships between adjacent skills and technologies, recognizing that proficiency in TensorFlow likely indicates knowledge of neural networks, even if not explicitly stated.
- Credential Verification: AI can validate certifications and qualifications against industry databases, highlighting verified credentials.
- Intelligent Shortlisting: Rather than producing binary "qualified/unqualified" determinations, advanced systems rank candidates based on comprehensive fit scoring across multiple dimensions.
Implementation Success Metrics:
Organizations implementing AI-powered screening report significant operational improvements:
- 58% reduction in time-to-screen per application
- 39% increase in qualified candidates progressing to interview stage
- 27% improvement in diversity of candidate pools
Best Practice for Implementation:
The most successful implementations maintain human oversight of AI recommendations. Recruiters should regularly audit a percentage of both accepted and rejected applications to continuously refine the system's parameters and ensure alignment with organizational needs.
2. Optimizing Job Descriptions for Maximum Effectiveness
Job descriptions represent an organization's first opportunity to attract qualified candidates—yet many suffer from unconscious bias, unclear requirements, or language that inadvertently discourages diverse applicants.
The Data on Description Deficiencies:
Analysis of over 76,000 job descriptions revealed that:
- 56% contained gender-coded language that disproportionately attracted one demographic
- 71% included requirements that were not actually necessary for success in the role
- 62% failed to clearly distinguish between essential and preferred qualifications
AI-Driven Improvements:
Advanced linguistic analysis tools now offer powerful capabilities to enhance job posting effectiveness:
- Inclusion Analysis: AI identifies potentially exclusionary language and suggests more neutral alternatives ("driven" instead of "aggressive," "experienced" instead of "expert").
- Readability Optimization: Algorithms assess description clarity, suggesting structural improvements for improved comprehension and engagement.
- Qualification Rationalization: AI helps organizations distinguish between true requirements and "nice-to-have" qualifications based on analysis of successful performers.
- Market Competitiveness Assessment: Systems benchmark descriptions against industry standards to ensure alignment with candidate expectations.
Documented Outcomes:
Organizations implementing AI-optimized job descriptions report:
- 41% increase in qualified applicants per posting
- 34% improvement in gender diversity among applicants
- 28% reduction in time-to-fill for hard-to-recruit positions
Implementation Framework:
For optimal results, organizations should establish clear guidelines for job description creation, including:
- Using standardized templates with AI-vetted language
- Separating "must-have" from "preferred" qualifications
- Focusing on responsibilities and outcomes rather than years of experience
- Including specific information about the team, culture and growth opportunities
3. Streamlining Candidate Communication and Scheduling
One of the most persistent challenges in recruitment involves coordinating communications and interview logistics across multiple stakeholders—a process that often creates friction and delays.
The Coordination Challenge:
Research indicates that the average time between initial application and first interview is 10-14 days, with each additional interview round adding 5-7 days to the hiring timeline. Much of this time is consumed by scheduling logistics and administrative coordination rather than substantive evaluation.
AI-Enabled Solutions:
Intelligent automation now offers sophisticated capabilities beyond basic scheduling tools:
- Conversational AI Interfaces: Advanced chatbots provide candidates with personalized information about the role, company culture, and hiring process—answering up to 78% of common questions without human intervention.
- Intelligent Scheduling: AI systems analyze interviewer availability, candidate preferences, and scheduling constraints to automatically propose optimal interview times and manage calendar coordination.
- Status Communication: Automated yet personalized updates keep candidates informed throughout the process, significantly improving engagement and reducing ghosting rates.
- Preparation Enhancement: AI tools can deliver role-specific preparation materials, company information, and interviewer backgrounds to candidates at appropriate intervals.
Measurable Impact:
Organizations implementing these technologies report:
- 62% reduction in scheduling-related administrative tasks
- 41% decrease in time-to-schedule interviews
- 36% improvement in candidate satisfaction scores
- 28% reduction in candidate ghosting/withdrawal rates
The Human Element:
While automation drives efficiency, successful implementations maintain appropriate personalization. The most effective approach involves:
- Using the candidate's name and specific role details in all communications
- Including brief personalized notes from the recruiter at key touchpoints
- Ensuring rapid human follow-up for complex questions
- Maintaining direct recruiter involvement for senior roles and specialized positions
4. Data-Driven Candidate Evaluation and Decision Support
Traditional hiring decisions often rely heavily on subjective impressions and unstructured feedback, creating inconsistency across hiring teams and increasing vulnerability to various cognitive biases.
The Evaluation Challenge:
Studies demonstrate that unstructured interviews have surprisingly low predictive validity (0.14 correlation with job performance) compared to structured assessments (0.51 correlation). Without standardized evaluation frameworks, hiring decisions frequently reflect interviewer bias rather than candidate capability.
AI-Enhanced Structured Assessment:
Advanced evaluation platforms now offer sophisticated capabilities:
- Multidimensional Assessment Frameworks: AI helps organizations develop comprehensive evaluation rubrics that assess technical skills, behavioral competencies, cultural alignment, and growth potential.
- Intelligent Scoring Models: Machine learning algorithms can weight evaluation criteria based on historical performance data, identifying which attributes truly predict success in specific roles.
- Comparative Analytics: Systems provide side-by-side comparison dashboards that normalize evaluator tendencies and highlight meaningful differences between candidates.
- Decision Support Tools: AI highlights evaluation gaps, inconsistencies between interviewers, and areas requiring additional assessment before final decisions.
Proven Results:
Organizations implementing data-driven evaluation report:
- 46% improvement in new hire performance ratings
- 38% reduction in early-stage attrition
- 41% increase in hiring manager satisfaction with recruitment outcomes
- 23% improvement in time-to-decision after final interviews
Implementation Best Practices:
For optimal results, organizations should:
- Develop clear, role-specific evaluation criteria before opening positions
- Require completion of structured feedback forms before hiring discussions
- Train interviewers on proper assessment techniques and bias awareness
- Regularly calibrate evaluation standards across teams
- Track correlation between hiring assessments and subsequent performance
5. Mitigating Bias Through Intelligent Process Design
Despite widespread commitment to diversity and inclusion, unconscious bias continues to influence hiring decisions across industries and organizational levels.
The Bias Challenge, Quantified:
Research demonstrates persistent disparities in recruitment outcomes:
- Candidates with ethnically identifiable names receive 50% fewer callbacks than identical resumes with majority-culture names
- Interview invitation rates drop by 45% for candidates above age 45, regardless of qualification
- Success predictions for identical work samples vary by up to 35% based on perceived gender of the creator
AI-Driven Approaches to Equity:
Advanced systems now offer practical approaches to reducing bias impact:
- Blind Screening Technology: AI facilitates anonymization of applications during initial review stages, removing names, graduation dates, and other potential bias triggers.
- Language Pattern Analysis: Sophisticated algorithms identify and flag potentially biased language in both job descriptions and candidate evaluations.
- Diversity Pipeline Monitoring: AI provides real-time analytics on demographic patterns throughout the recruitment funnel, highlighting stages where representation narrows.
- Alternative Credential Recognition: Machine learning models identify non-traditional indicators of capability, helping organizations recognize talent from diverse educational and professional backgrounds.
Measured Impact:
Organizations implementing bias-mitigation technologies report:
- 37% increase in gender diversity among technical hires
- 42% improvement in ethnic diversity of interview pools
- 29% expansion in age range of new hires
- 31% more hires from non-traditional educational backgrounds
Implementation Framework:
Effective bias mitigation requires integrating technology with organizational practices:
- Establish clear diversity targets for each stage of recruitment
- Implement structured interview processes with consistent questions
- Require diverse interview panels for all positions
- Regularly audit outcomes and adjust processes based on results
- Combine technological approaches with interviewer training and awareness programs
6. Predictive Analytics for Long-Term Hiring Success
Beyond identifying qualified candidates, organizations increasingly seek to predict which individuals will thrive and remain engaged over time—a capability now enhanced by sophisticated analytics.
The Prediction Challenge:
Traditional hiring approaches show limited ability to predict long-term outcomes:
- 46% of new hires fail within 18 months, despite passing standard interview processes
- Only 19% of organizations report high confidence in their ability to predict candidate success
- 34% of turnover occurs within the first year, suggesting significant misalignment in hiring decisions
AI-Enhanced Prediction Capabilities:
Advanced predictive technologies offer new approaches to long-term success forecasting:
- Success Pattern Identification: Machine learning algorithms analyze historical performance and retention data to identify behavioral and skill patterns associated with long-term success.
- Attrition Risk Modeling: AI can identify potential retention risks based on combination factors including role alignment, compensation equity, and career progression opportunities.
- Team Dynamics Analysis: Some systems assess how a candidate's working style and strengths complement existing team composition.
- Engagement Prediction: Sophisticated models predict cultural alignment and likely engagement levels based on values assessment and motivation analysis.
Documented Outcomes:
Organizations implementing predictive hiring analytics report:
- 37% reduction in first-year voluntary turnover
- 42% improvement in new hire performance ratings
- 33% increase in successful completion of onboarding milestones
- 29% enhancement in team effectiveness scores following new additions
Implementation Guidance:
For optimal results, organizations should:
- Establish clear definitions of "success" for each role and level
- Collect structured data on both performance and retention
- Regularly validate predictive models against actual outcomes
- Combine predictive insights with comprehensive onboarding support
- Use predictive data as one input among multiple factors in decision-making
Conclusion: The Future of AI-Enhanced Recruitment
The integration of artificial intelligence into the recruitment process represents not merely an operational enhancement but a fundamental transformation in how organizations identify, assess, and secure talent. When implemented thoughtfully, these technologies enable recruitment teams to:
- Process higher volumes of applications with greater consistency and reduced bias
- Identify qualified candidates who might be overlooked by traditional screening approaches
- Make more informed decisions based on comprehensive, structured data
- Provide enhanced candidate experiences through streamlined processes and improved communication
- Build more diverse teams by reducing the impact of unconscious bias
- Improve long-term hiring outcomes through data-driven prediction and selection
The most successful implementations maintain an appropriate balance between technological efficiency and human judgment. While AI excels at pattern recognition, data processing, and bias reduction, human recruiters remain essential for relationship building, nuanced assessment, and final decision-making.
As these technologies continue to evolve, organizations that develop integrated strategies—combining intelligent systems with skilled recruitment professionals—will gain significant competitive advantage in the ongoing competition for talent.
The future of recruitment is neither fully automated nor purely human-driven, but rather a thoughtful collaboration between artificial intelligence and human expertise—each complementing the other's capabilities to build stronger, more diverse, and more effective organizations.